Introduction#
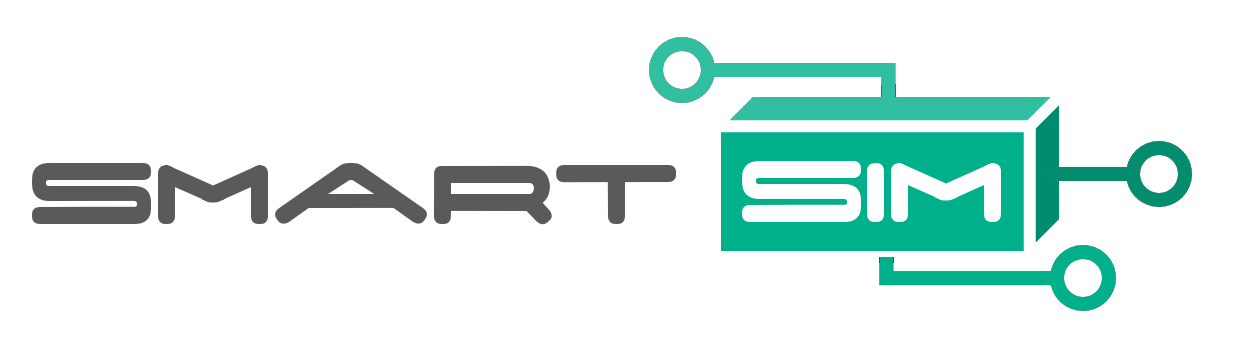
SmartSim enables scientists to utilize machine learning inside traditional HPC workloads
SmartSim provides this capability by
Automating the deployment of HPC workloads and distributed, in-memory storage (Redis).
Making TensorFlow, Pytorch, and ONNX callable from Fortran, C, and C++ simulations.
Providing flexible data communication and formats for hierarchical data, enabling online analysis, visualization, and processing of simulation data.
The main goal of SmartSim is to provide scientists a flexible, easy to use method for interacting at runtime with the data generated by simulation. The type of interaction is completely up to the user.
Embed calls to machine learning models inside a simulation
Create hooks to manually or programmatically steer a simulation
Visualize the progression of a simulation integration from a Jupyter notebook
The figure below shows the architecture of SmartSim for a given use case.
SmartSim can create, configure and launch workloads (called a Model
),
as well as groups of workloads (Ensembles
). The data communication between
a workload and in-memory storage is handled by the SmartRedis clients, available
in Fortran, C, C++, and Python.
Library Design#
There are two core components of SmartSim:
SmartSim (infrastructure library)
SmartRedis (client library)
The two libraries can either be used in conjunction or separately, depending on the needs of the user.
SmartSim (infrastructure library)#
The infrastructure library (IL) provides an API to automate the process of deploying HPC workloads alongside an in-memory database: Redis.
The key features of the IL are:
An API to start, monitor, and stop HPC jobs from Python or from a Jupyter notebook.
Automated deployment of in-memory data staging (Redis) and computational storage (RedisAI).
Programmatic launches of batch and in-allocation jobs on PBS, Slurm, and LSF systems.
Creating and configuring ensembles of workloads with isolated communication channels.
The IL can configure and launch batch jobs as well as jobs within interactive allocations. The IL integrates with workload managers, (like Slurm and PBS), if it is running on a supercomputer or cluster system.
The IL can deploy a distributed, shared-nothing, in-memory cluster of Redis
instances across multiple compute nodes of a supercomputer, cluster, or laptop.
In SmartSim, this clustered Redis deployment is called the Orchestrator
.
By coupling the Orchestrator with HPC applications, users can connect their workloads to other applications, like trained machine learning models, with the SmartRedis clients.
SmartRedis (Client Library)#
SmartRedis is a collection of Redis clients that support RedisAI capabilities and include additional features for high performance computing (HPC) applications. Key features of RedisAI that are supported by SmartRedis include:
A tensor data type in Redis
TensorFlow, TensorFlow Lite, Torch, and ONNXRuntime backends for model evaluations
TorchScript storage and evaluation
In addition to the RedisAI capabilities above, SmartRedis includes the following features developed for large, distributed HPC architectures:
Redis cluster support for RedisAI data types (tensors, models, and scripts).
Distributed model and script placement for parallel evaluation that maximizes hardware utilization and throughput
A
DataSet
storage format to aggregate multiple tensors and metadata into a single Redis cluster hash slot to prevent data scatter on Redis clusters and maintain contextual relationships between tensors. This is useful when clients produce tensors and metadata that are referenced or utilized together.An API for efficiently aggregating
DataSet
objects distributed on one or more database nodes.Compatibility with SmartSim ensemble capabilities to prevent key collisions with tensors,
DataSet
, models, and scripts when clients are part of an ensemble of applications.
SmartRedis provides clients in Python, C++, C, and Fortran. These clients have been written to provide a consistent API experience across languages, within the constraints of language capabilities. The table below summarizes the language standards for each client.
Language |
Version/Standard |
---|---|
Python |
3.9-3.11 |
C++ |
C++17 |
C |
C99 |
Fortran |
Fortran 2018 (GNU/Intel), 2003 (PGI/Nvidia) |